It is useful to see how digital noise works, so I contrived an image that shows noise in a fairly pure way, unlike what we see in actual digital photographs. Following is an example where I added Gaussian color noise uniformly across the image, without regard to color or luminance. From top to bottom, we have no added noise, then 25%, 50%, 100%, and then 200% noise at the bottom.
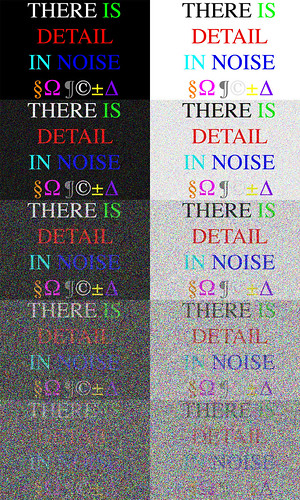
Viewing this, it is pretty obvious that noise reduces contrast, and that high contrast objects resist the effects of noise the best. The word ‘THERE’, — black on white or white on black — is recognizable even at the highest noise level, while blue on black becomes quickly unrecognizable. You can click on the image get a larger version.
But it is clear that there is noticeable detail even in severe noise. This consideration is important when devising methods to reduce noise, and when deciding how much noise to remove from an image.
When we view extremely noisy images, we are at risk of making two types of errors. We may perceive a signal in the noise when in fact nothing but noise actually exists: this is excess credulity and is called a “Type I error”. Likewise, we may not perceive a signal when in fact one does exist: this is excess skepticism and is a Type II error. Noise reduction software makes the same kind of decision: every pixel is judged as being some combination of signal or noise, and the final image produced may exhibit errors of both kinds. An excessively skeptical noise-reduction technique will generate images with very little noise, but with little detail also. An excessively credulous technique will pick out false detail, creating ugly artifacts.
In this sample image, a fluent reader of English would be able to pick out the words in the noise better than someone who is not familiar with English. I included the odd symbols at the bottom so I could include other colors, but it is also clear that familiarity with a symbol helps aid our recognition, and so these symbols seem to suffer particularly poorly under noise.
This example points out a severe limitation of noise reduction technology. We already know what the words are, since we are giving a noise-free example image at the top. Would we be able to determine the words if we are presented with only a noisy image? Our mind certainly ‘fills in’ missing information when we know for certain what that information must be. Furthermore, natural languages have much redundancy in grammar and spelling, and so this helps us to identify noisy unknown sentences if we are familiar with the language.
Noise reduction therefore quickly becomes an ‘AI-complete’ problem — that is, it requires a computer with full human intelligence. If we insist that our noise-reduction algorithm be perfect, then we are asking the computer to have the knowledge of God. Rather, we must be humble and instead accept general-purpose solutions that reduce enough noise to produce an image that is good enough; or, we must accept that our image is too noisy and instead re-shoot the subject using better equipment or technique.
There are special-purpose noise reduction algorithms for character recognition; these take into account the shapes of letters, common dictionary words, and basic grammar. These algorithms will allow a computer to scan and read a book, but these will be completely unsuitable for general photography.
Fortunately, the image above is quite contrived, and the noise I added does not have the characteristics that we find in a digital photo. I provided that image for the purpose of demonstrating the effects of noise in general, without the complications found in a photograph. However, I would challenge any interested reader to download that image, and try various noise-reduction techniques on it. I failed to significantly reduce the worst noise using various techniques — and ugly digital artifacts or side-effects of these attempts are quite obvious:
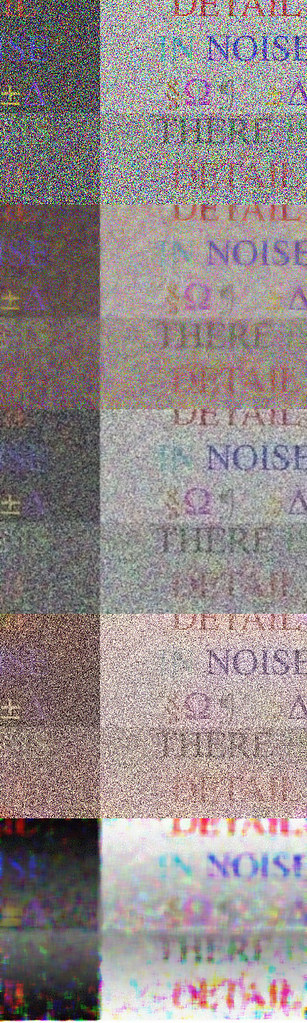
From top to bottom are: a section from the original image; the same section processed with Photoshop Reduce Noise; then Noiseware Professional; Surface Blur of chroma channels; and finally the popular Dust and Scratches noise reduction from Photoshop. Note the color shifts and poor performance of all methods. Are any of these results better than then original noisy image? It seems not.
Following is a generated image that has a noise profile closer to what we find in natural photographs. Here, noise is great in the shadows, and is slight in the highlights. There are different levels of overall noise in each channel, similar to what is found with photos taken under incandescent illumination: green has the least noise, red a bit more, and blue has much more noise.
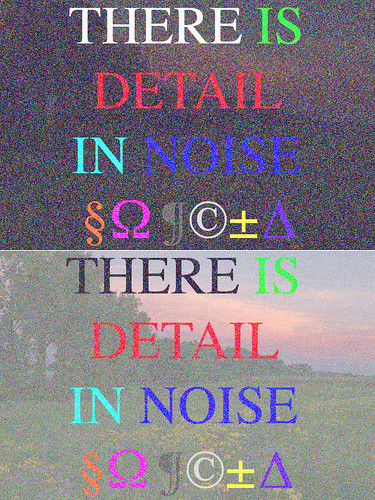
The default settings of Noiseware Professional does a decent job of denoising this image, and it could be easily cleaned up nicely with a bit more effort:
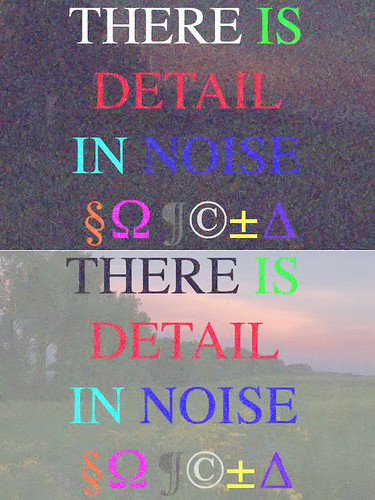
The limits of noise-reduction technology tell us that we have to be careful to avoid noise when taking a picture, and that we have to use intelligence when applying noise reduction, and not to fully rely on the computer. If we know for certain what the signal ought to be, then we can do manual retouching: in the image above, I would retouch the blue-on-black word NOISE, and do general blurring of the dark background. If our job is to produce a good image, and not necessarily a faithful one, then artistic judgment is needed to create plausible detail with little noise.
No comments:
Post a Comment